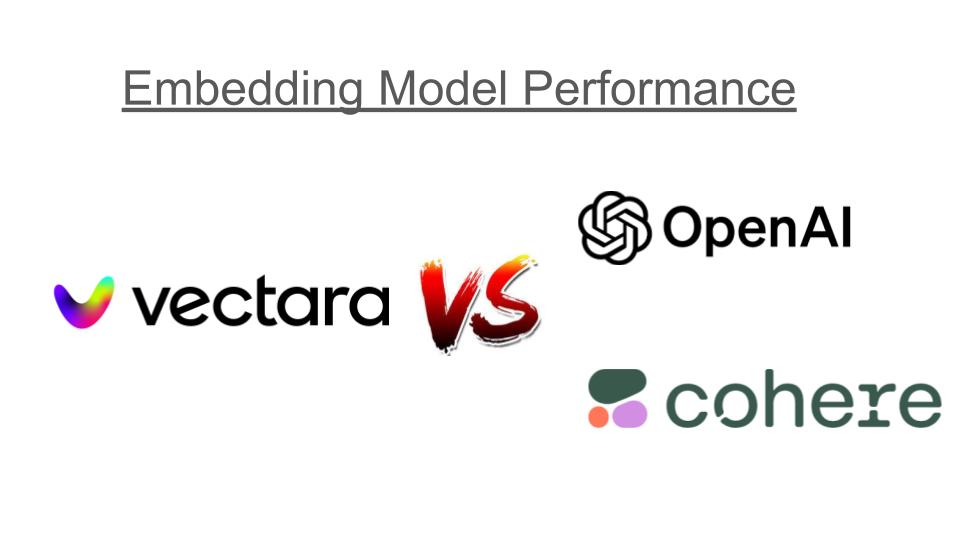
Application Development
The Latest Benchmark Between Vectara, OpenAI and Cohere’s Embedding Models
Vectara’s Boomerang stands out as the optimal choice for production use cases, balancing precision, embedding size, and storage costs effectively.
June 13 , 2024 by Nick Ma & Vivek Sourabh
Introduction
Boomerang is Vectara’s leading embedding model launched in September 2023, that is small yet powerful, and designed for production use cases.
OpenAI released its latest embedding models, OpenAI text-embedding-3-small and OpenAI text-embedding-3-large, in January 2024.
Cohere released its latest embedding models, Cohere Embed v3 light and Cohere Embed v3, in November 2023.
In this article, we compare Boomerang with the latest embeddings from OpenAI and Cohere, to see how Boomerang holds against the competition.
Comparing Boomerang’s “Ability to Rank”
We first look into comparing different models on quality: their ability to rank text chunks or passages against a given query.
The key metrics we benchmarked are Mean Reciprocal Rank (MRR) and Mean Average Precision (MAP). MAP evaluates overall ranking quality, while MRR prioritizes finding the first (most relevant) match.
English Language Performance
In addition to the BEIR benchmark presented in the introductory Boomerang blog post, we performed a fresh round of evaluation with the SQuAD and SQuAD shift benchmarks (See Figure 1), using MRR as the key metric.
Key insights include:
- Boomerang is roughly equivalent to OpenAI text-embedding-3-small and slightly behind OpenAI text-embedding-3-large.
- Boomerang is roughly equivalent to Cohere embed v3 light and slightly behind Cohere v3.
Figure 1: SQuAD and SQuAD Shifts
Multilingual and Cross-lingual Performance
In addition to the MIRACLE benchmark mentioned in the introductory Boomerang blog post, we re-ran the XQuAD-R benchmark (See Figure 2) with the latest OpenAI and Cohere models
Key takeaways are:
- Boomerang is far superior to OpenAI text-embedding-3-small and significantly better than OpenAI text-embedding-3-large.
- Boomerang outperforms Cohere v3 light and is slightly better than Cohere v3.
Figure 2: XQuAD-R
To sum it up – here is the TL,DR: Vectara’s Boomerang is on par with OpenAI and Cohere on English performance and significantly better on multilingual and cross-lingual performance.
Comparing Embedding Size and Storage Costs
When selecting models for production use cases, it is crucial to balance precision, embedding size, and storage costs. Prioritizing only precision may result in a highly accurate but expensive model to run, leading to trade-offs in both costs and latency.
The table below shows the embedding size of different models.
Model Name |
Embedding Size |
Boomerang |
768 |
Cohere V3 Light |
384 |
Cohere V3 |
1024 |
OpenAI text-embedding-3-small |
1536 |
OpenAI text-embedding-3-large |
3072 |
The memory required to store embeddings from each model is directly proportional to the embedding size. Consequently, storing OpenAI’s text-embedding-3-large will cost 8 times more than Cohere V3 light and 4 times more than Boomerang.
Success for us means achieving a small embedding model (<1K) with high precision and low storage costs. Among the models considered, only Boomerang and Cohere V3/V3 light meet these criteria.
Comparison of Boomerang with Cohere V3 Light and Cohere V3
- Precision: For multilingual and cross-lingual datasets, Boomerang outperforms.
- Embedding Size: Cohere V3 light is the smallest, followed by Boomerang and Cohere V3.
- Storage Costs: Storage costs align with embedding sizes—Cohere V3 light incurs the least cost, followed by Boomerang and Cohere V3.
Figure 3: Trade-Off Between Quality and Embedding Size
The TL;DR in this case: Boomerang stands out as the optimal choice for production use cases, effectively balancing precision, embedding size, and storage costs.
Want to Try Out Boomerang? Sign Up for an Account and Connect With Us!
As always, we’d love to hear your feedback! Connect with us on our forums or on our Discord. Sign up for a free account to see how Vectara can help you easily leverage retrieval-augmented generation in your GenAI apps.